This Week in AI: OpenAI finds a partner in higher ed
Keeping up with an industry as fast-moving as AI is a tall order. So until an AI can do it for you, here’s a handy roundup of recent stories in the world of machine learning, along with notable research and experiments we didn’t cover on their own.
This week in AI, OpenAI signed up its first higher education customer: Arizona State University.
ASU will collaborate with OpenAI to bring ChatGPT, OpenAI’s AI-powered chatbot, to the university’s researchers, staff and faculty — running an open challenge in February to invite faculty and staff to submit ideas for ways to use ChatGPT.
The OpenAI-ASU deal illustrates the shifting opinions around AI in education as the tech advances faster than curriculums can keep up. Last summer, schools and colleges rushed to ban ChatGPT over plagiarism and misinformation fears. Since then, some have reversed their bans, while others have begun hosting workshops on GenAI tools and their potential for learning.
The debate over the role of GenAI in education isn’t likely to be settled anytime soon. But — for what it’s worth — I find myself increasingly in the camp of supporters.
Yes, GenAI is a poor summarizer. It’s biased and toxic. It makes stuff up. But it can also be used for good.
Consider how a tool like ChatGPT might help students struggling with a homework assignment. It could explain a math problem step-by-step or generate an essay outline. Or it could surface the answer to a question that’d take far longer to Google.
Now, there’s reasonable concerns over cheating — or at least what might be considered cheating within the confines of today’s curriculums. I’ve anecdotally heard of students, particularly students in college, using ChatGPT to write large chunks of papers and essay questions on take-home tests.
This isn’t a new problem — paid essay-writing services have been around for ages. But ChatGPT dramatically lowers the barrier to entry, some educators argue.
There’s evidence to suggest that these fears are overblown. But setting that aside for a moment, I say we step back and consider what drives students to cheat in the first place. Students are often rewarded for grades, not effort or understanding. The incentive structure’s warped. Is it any wonder, then, that kids view school assignments as boxes to check rather than opportunities to learn?
So let students have GenAI — and let educators pilot ways to leverage this new tech to reach students where they are. I don’t have much hope for drastic education reform. But perhaps GenAI will serve as a launchpad for lesson plans that get kids excited about subjects they never would’ve explored previously.
Here are some other AI stories of note from the past few days:
Microsoft’s reading tutor: Microsoft this week made Reading Coach, its AI tool that provides learners with personalized reading practice, available at no cost to anyone with a Microsoft account.
Algorithmic transparency in music: EU regulators are calling for laws to force greater algorithmic transparency from music streaming platforms. They also want to tackle AI-generated music — and deepfakes.
NASA’s robots: NASA recently showed off a self-assembling robotic structure that, Devin writes, might just become a crucial part of moving off-planet.
Samsung Galaxy, now AI-powered: At Samsung’s Galaxy S24 launch event, the company pitched the various ways that AI could improve the smartphone experience, including through live translation for calls, suggested replies and actions and a new way to Google search using gestures.
DeepMind’s geometry solver: DeepMind, the Google AI R&D lab, this week unveiled AlphaGeometry, an AI system that the lab claims can solve as many geometry problems as the average International Mathematical Olympiad gold medalist.
OpenAI and crowdsourcing: In other OpenAI news, the startup is forming a new team, Collective Alignment, to implement ideas from the public about how to ensure its future AI models “align to the values of humanity.” At the same time, it’s changing its policy to allow military applications of its tech. (Talk about mixed messaging.)
A Pro plan for Copilot: Microsoft has launched a consumer-focused paid plan for Copilot, the umbrella brand for its portfolio of AI-powered, content-generating technologies, and loosened the eligibility requirements for enterprise-level Copilot offerings. It’s also launched new features for free users, including a Copilot smartphone app.
Deceptive models: Most humans learn the skill of deceiving other humans. So can AI models learn the same? Yes, the answer seems — and terrifyingly, they’re exceptionally good at it. according to a new study from AI startup Anthropic.
Tesla’s staged robotics demo: Elon Musk’s Optimus humanoid robot from Tesla is doing more stuff — this time folding a t-shirt on a table in a development facility. But as it turns out, the robot’s anything but autonomous at the present stage.
More machine learnings
One of the things holding back broader applications of things like AI-powered satellite analysis is the necessity of training models to recognize what may be a fairly esoteric shape or concept. Identifying the outline of a building: easy. Identifying debris fields after flooding: not so easy! Swiss researchers at EPFL are hoping to make it easier to do this with a program they call METEOR.
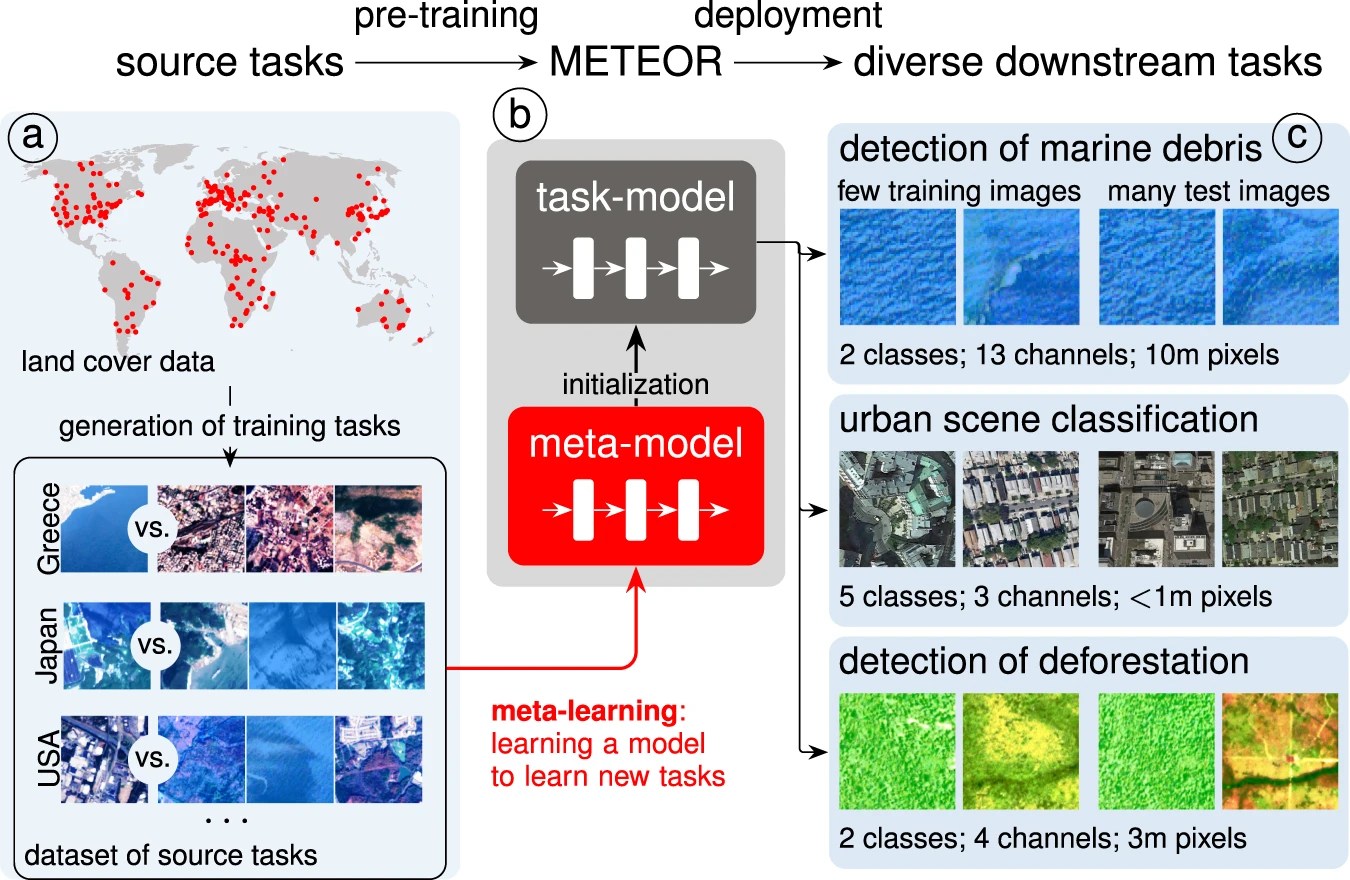
Image Credits: EPFL
“The problem in environmental science is that it’s often impossible to obtain a big enough dataset to train AI programs for our research needs,” said Marc Rußwurm, one of the project’s leaders. Their new structure for training allows a recognition algorithm to be trained for a new task with just four or five representative images. The results are comparable to models trained on far more data. Their plan is to graduate the system from lab to product with a UI for ordinary people (that is to say, non-AI-specialist researchers) to use it. You can read the paper they published here.
Going the other direction — creating imagery — is a field of intense research, since doing it efficiently could reduce the computation load for generative AI platforms. The most common method is called diffusion, which gradually refines a pure noise source into a target image. Los Alamos National Lab has a new approach they call Blackout Diffusion, which instead starts from a pure black image.
That removes the need for noise to begin with, but the real advance is in the framework taking place in “discrete spaces” rather than continuous, greatly reducing the computational load. They say it performs well, and at lower cost, but it’s definitely far from wide release. I’m not qualified to evaluate the effectiveness of this approach (the math is far beyond me) but national labs don’t tend to hype up something like this without reason. I’ll ask the researchers for more info.
AI models are sprouting up all over the natural sciences, where their ability to sift signal out of noise both produces new insights and saves money on grad student data entry hours.
Australia is applying Pano AI’s wildfire detection tech to its “Green Triangle,” a major forestry region. Love to see startups being put to use like this — not only could it help prevent fires, but it produces valuable data for forestry and natural resource authorities. Every minute counts with wildfires (or bushfires, as they call them down there), so early notifications could be the difference between tens and thousands of acres of damage.

Permafrost reduction as measured by the old model, left, and the new model, right.
Los Alamos gets a second mention (I just realized as I go over my notes) since they’re also working on a new AI model for estimating the decline of permafrost. Existing models for this have a low resolution, predicting permafrost levels in chunks about 1/3 of a square mile. That’s certainly useful, but with more detail you get less misleading results for areas that might look like 100% permafrost at the larger scale but are clearly less than that when you look closer. As climate change progresses, these measurements need to be exact!
Biologists are finding interesting ways to test and use AI or AI-adjacent models in the many sub-fields of that domain. At a recent conference written up by my pals at GeekWire, tools to track zebras, insects, even individual cells were being shown off in poster sessions.
And on the physics side and chemistry side, Argonne NL researchers are looking at how best to package hydrogen for use as fuel. Free hydrogen is notoriously difficult to contain and control, so binding it to a special helper molecule keeps it tame. The problem is hydrogen binds to pretty much everything, so there are billions and billions of possibilities for helper molecules. But sorting through huge sets of data is a machine learning specialty.
““We were looking for organic liquid molecules that hold on to hydrogen for a long time, but not so strongly that they could not be easily removed on demand,” said the project’s Hassan Harb. Their system sorted through 160 billion molecules, and by using an AI screening method they were able to look through 3 million a second — so the whole final process took about half a day. (Of course, they were using quite a large supercomputer.) They identified 41 of the best candidates, which is a piddling number for the experimental crew to test in the lab. Hopefully they find something useful — I don’t want to have to deal with hydrogen leaks in my next car.
To end on a word of caution, though: a study in Science found that machine learning models used to predict how patients would respond to certain treatments was highly accurate… within the sample group they were trained on. In other cases, they basically didn’t help at all. This doesn’t mean they shouldn’t be used, but it supports what a lot of people in the business have been saying: AI isn’t a silver bullet, and it must be tested thoroughly in every new population and application it is applied to.